

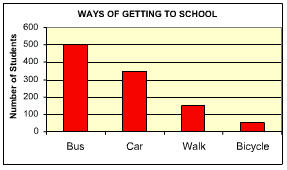
A versatile naming scheme that is consistent across papers and contains the relevant information the user desires will make digitising and sharing much easier if thought through carefully before starting a project. However, it is important for users to think carefully about reproducibility at this stage. Nonetheless, how users set up their directory is really up to them. Even if only figures from a single paper are digitised, one paper at a time, an overall figure directory will work perfectly because metaDigitise() will only cycle through incomplete figures, so figures can be added at anytime. The above directory structure is probably the easiest in combination with a clear and unambiguous naming scheme for each figure. For example the directory structure could look like: * Main project directory This cuts out the need to change directories constantly. This could be done by simply setting up a file structure as follows and then using metaDigitise() with path names (i.e., the directory) for each papers folder: * Main project directoryĪn alternative directory structure (and probably the most flexible) would be to simply have a set of different figures with an informative and relevant naming scheme to make it easy to identify the paper and figure the data come from. For example, one might have 3–4 figures from a single paper that need extracting and the user may want to focus on a single paper at a time while the information about a paper is on hand. However, users can get creative in how they set up the directories of figures to facilitate extraction.
DATATHIEF FOR BAR GRAPHS PDF
pdf images can be used) from many different papers and that are of different types. MetaDigitise() can work on a directory with figures (currently. The data from completed figures will automatically be written to the caldat/ folder for later use and editing, should the user need to do this. Users can stop mid-way through a folder by simply exiting after the last plot they have digitised. This information is then all stored in a data frame or list at the end of the process, saving quite a bit of time. metaDigitise essentially will bring up each figure within a folder automatically and allow the user to click and enter the relevant information about a figure as they go. This is useful because it expedites digitising figures as it prevents users from having to constantly specify the directories and / or paths where files are stored. metaDigitise will also handle these situations seamlessly by simply cycling through all figures within a directory. However, often many figures need extracting from a single paper or set of papers.

Users can extract single figures (if this is all they have) using the metaDigitise() function with a path name to the directory with the file. The metaDigitise package is quite flexible.
DATATHIEF FOR BAR GRAPHS UPDATE
This makes sharing figure digitisation and reproducing the work of others simple and easy and allows meta-analysts to update existing studies more easily. It has functions that allow users to redraw their digitisations on figures, correct anything and access the raw calibration data which is written automatically for each figure that is digitised into a special caldat folder within the directory. metaDigitise has also been built for reproducibility in mind. This makes it easy to add new figures at anytime. Conveniently, when needing to process many figures at different times metaDigitise will only import figures not already completed within a directory. Summaries will condense multiple figures into data frames or lists (depending on the type of figure) and these objects can easily be exported from R, or if using the raw data, analysed in any way the user desires. It also provides users with options to conduct the necessary calculations on raw data immediately after extraction so that comparable summary statistics can be obtained quickly. metaDigitise allows users to extract information from a figure or set of figures all within the R environment making data extraction, analysis and export more streamlined. Often third party applications are used to do this (e.g., graphClick or dataThief), but the output from these are handled separately from the analysis package, making this process more laborious than it needs to be given that resulting output still requires substantial downstream processing to acquire the relevant statistics of interest. MetaDigitise is an R package that provides functions for extracting raw data and summary statistics from figures in primary research papers.
